How Much Do Data Analytics Services Cost?
(UPDATE)
CLICK HERE TO SEE ACTUAL PRICING
The post below is very detailed and will definitely get your head around all the pricing factors for data analytics. But if you just want a click glance at pricing, check out that link above for a quick TL;DR explanation of our industry-leading, transparent, fixed-fee pricing, including a video showing our price-book.
Data Analytics Pricing (long read)
Small and mid-sized businesses invest anywhere from $40,000 to hundreds of thousands of dollars annually for data analytics. This includes paying for people as well as technology.
Until now, the best information for SMBs was from an informal survey and analysis from 2020 that are severely lacking. They only considered reporting tools, completely ignored people costs, and the respondents were limited to mostly IT specialists.
This article is to share actual numbers we see day in and day out from interacting with prospective buyers, customers, partners, vendors, and others in the modern data industry. We will explain the factors that influence the cost of data analytics and how you can design a system that brings the most value to your investment.
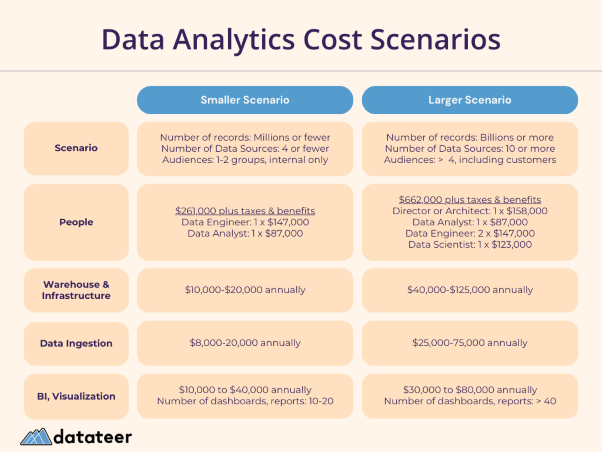
In modern business, data analytics is a necessity. It's a powerful tool that helps businesses make informed decisions, understand customer behavior, and predict market trends. It can even create opportunities for improved products and new revenue. But, as with most powerful tools, there's a data analytics cost involved. Understanding these costs is crucial for any business looking to harness the power of data analytics.
In this article, we will explore not only the costs but also how to assess the tradeoffs and maximize your return on investment.
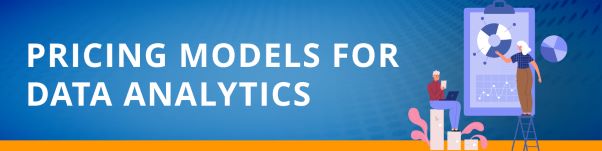
Data Analytics Pricing Models
In this section we will break down common pricing models. For a fuller explanation of how to evaluate data analytics consultants, including pricing, check out How to Evaluate and Hire a Data Analytics Consultant.
In-House Analytics Team
For a small or medium-sized business, in-house teams, and platforms are often the most expensive option. They can grant the most control and teams can get very deep in the domain and industry expertise of a business.
Salary and benefits expectations are increasing rapidly. The average Data Engineer salary in the US is reportedly $146,762 (source). Other specialized roles are also increasing and are shown below. Building a full team can easily be $500,000 to $1,000,000 per year, and that does not include platform and tool costs.
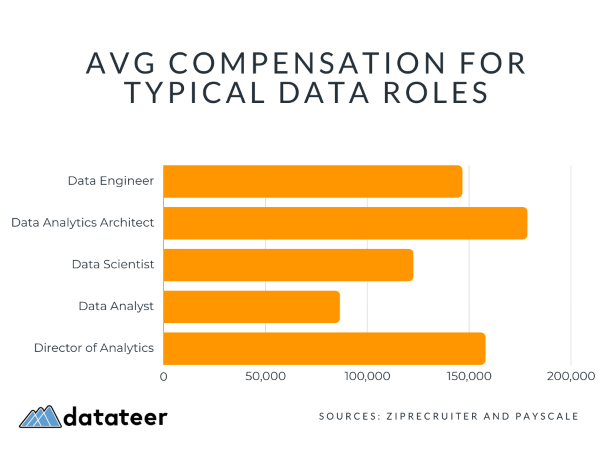
Consulting Hourly Rates or Retainers for Analytics Agencies & Freelancers
External consultants or freelancers often charge by the hour. Typically you get what you pay for, and finding the right consultant or freelancer can be just as difficult as recruiting the right employee.
When considering analytics consulting, it's important to note that pricing can be quite variable. Hourly rates can range from $80 an hour to $300 an hour. Junior staff or people with limited experience may charge less. Companies with foreign staff or freelancers from places like India or Eastern Europe may charge as little as $25 per hour.
Foreigners may be out of the question due to data regulations applicable to your company. Results are often mixed, with time zone, cultural, and language barriers.
Some consultants offer a retainer model. This is a nice option to help with budgeting and to maintain costs. In this situation, you and the consultant are limiting the scope or spreading it out over a longer period of time to stay within the time available in the negotiated retainer. Almost always, the retainer is based on an underlying hourly rate, so you can ask what that internal rate is to compare with other options.
- Example 1: Data Extractions
- One-time costs. Example costs for setting up a data source can range from just a few hours of work to configure a pre-built data extractor, to tens of thousands of dollars to create a custom-built data extractor.
- Ongoing costs. Ongoing costs for small amounts of data for a data source that needs no support can be less than $200/month. For large amounts of data or unreliable data sources, costs can exceed $10,000 per month. For big data such as terabytes of data per month, costs can grow to high five figures
- Example 2: Dashboard with Charts and Graphs
- One-time costs. Dashboards can vary widely based on the number of audiences, metrics, and visualizations included. A simple dashboard with underlying data ready to go can be implemented in just a few hours. Further refinements on a simple dashboard, or a complex dashboard, can cause costs to increase. Dashboards that also need new metrics or new data sources will take more to build out as well. The largest dashboards can be projects themselves, up to $15,000.
- Ongoing costs. Dashboards are built-in tools that have ongoing license or subscription fees. Apart from this, dashboards themselves are usually maintenance-free. However, they rely on underlying metrics, data pipelines, and data sources. With so many moving parts, the data in the dashboard can be impacted and require support or troubleshooting. Setting up monitoring will ensure the data remains accurate.
Fixed-Price Projects
Some consultants and freelancers offer fixed-price projects or price-per-deliverables. In theory, this shifts the risk of scope increases and cost overruns from the purchaser to the seller. This can be a nice arrangement because the consultant is incentivized to finish the project within a certain price.
Because of this shift in risk, fixed-price projects are often charged at a premium over projects done for an hourly rate.
This model has some things to watch out for or manage. The same incentives a consultant has to finish the project below a certain price will also incentivize behavior to reduce quality, negotiate a reduction in scope, or require change orders for any modifications to scope, even if they should have been factored in originally.
Data Assets under Management
Datateer’s Managed Analytics has a pricing model based on how many Data Assets we manage for our customers. The support and data analytics platform, as well as all the underlying technical components (extractions, schedule, transformations, usage) are all included.
This pricing model allows for planning around the business concepts of Data Assets instead of around technical components
See more details on Datateer’s Managed Analytics pricing model here or read more about Analytics as a Service here.
Data Consumption
Data infrastructure and modern data warehouses are based on cloud infrastructure such as Amazon AWS, Google GCP, or Microsoft Azure. Because of this, they often have a consumption price model. Snowflake, BigQuery, and Synapse all have variations of a consumption model.
The more data you have, the more you process it, and the more you query it all drive up costs. And instead of measuring consumption in straight currency, these vendors often have a proprietary system of “credits” that eventually translates into currency like dollars. This all makes accuracy of cost projections extremely difficult.
Data extraction vendors such as Fivetran also have a consumption-based model for the same reasons. Similarly, the more data you move, the higher the bill.
All these vendors have various calculators to try and help you project costs, and monitoring tools to manage costs if they spike or are higher than anticipated.
User Seats
Other products, especially business intelligence, visualization, and analysis tools, are typical per-seat pricing models. Often there is also a base or platform fee to ensure a minimum price threshold. Lower-end BI tools can start at $10 per month per user. More capable tools can charge $1,000 per month per user.
Other tools, such as orchestration/scheduling, monitoring, observability, and transformations, have consumption pricing, seat pricing, or a combination of the two.
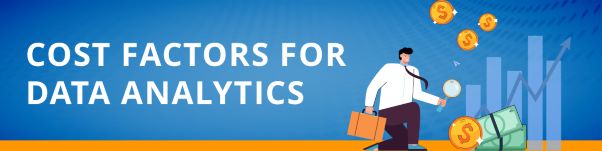
Key Factors Influencing Data Analytics Costs
Navigating the costs of data analytics can be complex, because there is no one-size-fits-all solution, and there are many components involved to make a complete solution. Understanding each of these factors will help you create a solution that works best for your business, and maximize your return on investment.
Any general implementation project success factors such as establishing clear goals and support from top management obviously apply here, as they do for any initiative.
Here are the factors that are specific to data analytics:
- Number of data sources. A data source is any database, operational system, SaaS product, API, etc that provides data for analysis. Each data source is a potential point of failure and a connection that must be configured, scheduled, and managed. Data coming from a data source must be cataloged, cleansed, and otherwise prepared for use in analytics.
- Number of metrics. A metric is a calculation or measure that can be analyzed to contribute to a business decision or answer a question. Metrics can be complex if they are composed of data from multiple data sources. Even if a metric is simple, it can drive debate and discussion about the correct business definition, as a metric can drive behaviors in a business’s operations.
- Number of data products. A data product is any output from data analytics processes like reports, dashboards, or data models. Each product requires resources for development, maintenance, and updates. More products mean more time spent on design, implementation, and quality assurance.
- Size of data. This refers to the volume of data being analyzed. Larger datasets require more storage, processing power, and potentially more sophisticated tools and procedures, all of which contribute to higher costs.
- Complexity of data. Complex data might involve various formats, unstructured data, or data that requires extensive transformation. High complexity increases the time and expertise needed for analysis, thus impacting costs. When issues occur in the data processing, complex data requires more support and troubleshooting effort.
- Quality of data. High-quality data is easier and cheaper to analyze. Poor quality data, which may be inaccurate, incomplete, or inconsistent, requires additional effort in cleaning and preparation, thus increasing costs. Lower-quality data can come from manual data entry, loose process controls, or loosely defined business processes.
- Customer-facing vs internal audiences. Data analytics intended for customer-facing applications, like personalized marketing or customer-facing data products, might require more stringent accuracy, security, and design standards compared to internal use cases. Of course, this depends on your needs, but generally externally facing analytics have higher standards than data products targeting internal audiences.
- Infrastructure and tools. Data analytics, like any solution based on a foundation of technology, is notorious for having a large number of products and tools. The more products and tools require not only license and usage costs but also additional maintenance and training burden on staff.
- Freshness requirements. The frequency at which data needs to be updated or refreshed impacts costs. Real-time or near-real-time data analysis requires more sophisticated, often more expensive technology and infrastructure compared to less frequent updates.
- Number of audiences. Different audiences (e.g., executive leadership, operations staff, customers) may require customized views or interpretations of the data, increasing the complexity and cost of data analytics projects.
- Number of subject areas. The range of topics or business areas covered by data analytics (like sales, marketing, and operations) can affect costs. More subject areas typically mean a broader range of data types and sources, increasing the complexity and cost.
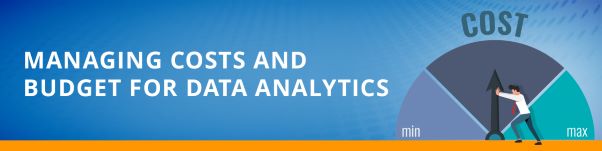
How to Manage Costs and Budget for Data Analytics
Evaluating and managing the various factors that influence the cost of data analytics is key to maximizing ROI.
By following the strategies below, you can effectively manage the key cost factors in data analytics and maximize the return on your investment.
Prioritize your business goals or outcomes
The potential value of data already available to your business is exciting and often gets executives and stakeholders thinking big. Explicitly define possible goals and outcomes and prioritize them or put them into phases.
For example, one common goal is to create a customer-facing set of dashboards to allow customers to have self-service access to real-time information anytime they want. If this is the top priority, it can limit the number of Data Sources and Metrics that are in scope for the first milestone.
Simplify Metrics and KPIs
Organizations that are getting started in data analytics and do not yet have a culture of data-informed decision-making often have a long list of potential metrics they want to collect and KPIs they want to use.
By limiting the number of metrics and KPIs or simplifying their definitions, you can significantly reduce the scope of implementation and cost of maintenance. Often, a handful of key metrics can make a big impact to begin transforming the culture of an entire organization.
Reduce the Number of Data Assets, Audiences, and Subject Areas
Each Data Source, Metric, and Data Product requires analysis, design, implementation, maintenance, training, and support. Each Audience and subject area expands the scope and therefore cost of the data analytics program.
By simply forcing a reduction in the number of Data Assets, Audiences, and subject areas, the cost naturally decreases. This is not only from a reduction in scope but also from a reduction in complexity.
Regularly Review and Purge
Regular reviews of your data analytics assets are essential. This process involves assessing the ongoing relevance and value of each data source, metric, and data product. Over time, some assets may become outdated or redundant. By periodically purging these unnecessary elements, you can streamline your analytics processes, reduce costs, and maintain a sharp focus on what delivers the most value to your organization.
Be Realistic about Timeliness Requirement
It's important to balance the need for up-to-date data with the costs associated with achieving real-time or near-real-time analytics. Assess the actual business need for the freshness of data. In many cases, slightly less current data can be sufficient and far more cost-effective. Tailoring the timeliness of your data analytics to the real needs of the business can lead to significant savings.
Minimize Tools and Simplify Data Architecture
Opt for a lean approach to tools and technology. Use a minimal set of tools that adequately meet your needs, rather than a complex array of overlapping technologies. This not only cuts down on costs but also simplifies your data architecture, making it easier to manage and maintain. Embracing integrated tools that can handle multiple functions can also be a cost-effective strategy.
Change Management, Training, and Education
Implementing and managing a data analytics strategy is as much about people as it is about technology. Invest in change management to help your team adapt to new tools and processes. Also, focus on training and education to build a data-informed culture. This investment in your people can maximize the usage and effectiveness of your analytics tools, leading to better decision-making and a higher ROI.
By following these strategies, you can effectively manage the key cost factors in data analytics and maximize the return on your investment. This approach ensures that your data analytics initiative is not only cost-efficient but also aligned with your business goals and capable of providing actionable insights.
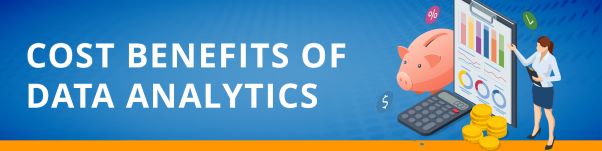
Data Analytics Cost-Benefit Analysis
When businesses invest in data analytics, they’re essentially planting seeds for future growth. The ROI of data analytics can be substantial, but it's not always immediate. It's about playing the long game. By analyzing large sets of data, companies can uncover hidden patterns, market trends, customer preferences, and other valuable insights. These insights can lead to more informed decision-making, efficiency improvements, enhanced customer experiences, and new revenue opportunities.
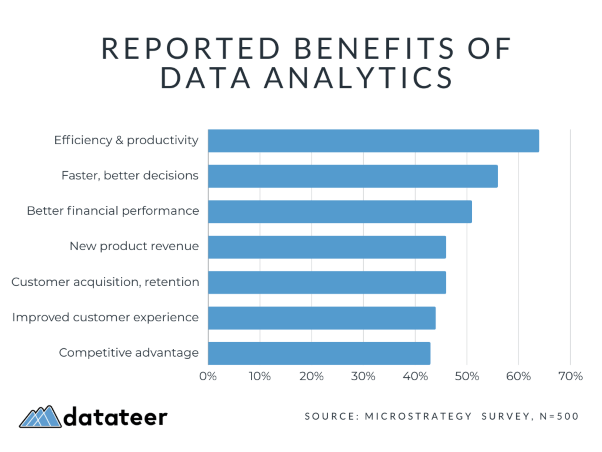
Here are some real-world examples of ways companies have looked at the data analytics cost-benefit analysis:
Efficiency & Productivity
Kelsey Waters, Senior Director of Operations at Equinix, says, “We were data rich but information poor.” They had several analysts compiling reports for two weeks at every month's end. This was completely time-consuming and error-prone. By setting up an automated way to aggregate data and calculate metrics, their reports are now nearly real-time.
In addition to the benefits of accurate and timely reporting, their analysts are now free to spend their time analyzing the data and answering questions–instead of wrangling data to compile reports.
See other ways data analytics can lower costs.
Faster, Better Decisions
Project Broadcast tracks numerous metrics around their customers’ use of their messaging platform. By seeing daily updates to usage patterns, especially in response to changes in functionality or customer outreach efforts, they can optimize and fine-tune their customer experience. Says CEO Jake Dempsey, "I use the dashboards every day! Huge ROI"
New Product Revenue
Portland Cement Association has a dataset about manufacturing and distribution trends in the manufacturing industry that is unique, and valuable for their member organizations. In the past, they would publish a quarterly newsletter and PDF report to update their members on these trends.
Today, they have self-service dashboards that all of their members can access on demand. Members get more timely updates, and Portland Cement Association no longer has to manually compile these insights. Plus, they have turned this into a new revenue stream.
Customer Acquisition & Retention, Revenue Expansion
By analyzing trends in customer behavior, Equinix Metal is able to accurately predict customers who are ready for an upgrade–and those showing signs of potential churn.
Account managers can now be targeted and prescriptive with their time. Instead of blindly reaching out to all accounts equally, they can prepare and spend more time on these customers who are ready for more engagement. The result has been significantly increased revenue expansion and reduced churn.
Competitive Advantage
Herrmann Global has deep profile and personality data from administering 2 million assessments over the last 30 years. By adding self-service reports into products, they now have a completely differentiated customer experience that sets them apart from their competitors.
In another example, Appcast is now able to do something other job advertising companies cannot. Most agencies have to manually compile advertising data from various advertising channels–Google, Facebook, TikTok, etc. By automatically tying campaigns together across channels, Appcast now has a unique ability to share with their customers performance insights, unified across channels.
Summary
In summary, figuring out the cost of data analytics is complex but crucial for businesses. It's not just about how much you pay, but also about getting good value for your money. Whether you have an in-house team or hire outside experts, the costs vary. Remember, cheaper options might not always offer the best value. Investing in data analytics can lead to better decisions and more money made in the long run.
By being smart about your goals, choosing the right tools, and training your team well, you can keep costs under control and make the most out of your data analytics. This approach is key for businesses that want to grow and stay ahead in today's data-driven world.